Among the many existing numerical weather prediction (NWP) models, the Weather Research and Forecasting (WRF) model is the most widely used open-source predictive model for weather forecasting worldwide. The recently developed WRF-Solar Ensemble Prediction System (EPS) allows us to provide high quality probabilistic solar irradiance forecasts.
What is WRF-Solar?
As more and more photovoltaic (PV) systems are being installed around the Globe, intraday and day-ahead solar power forecasting becomes increasingly important. In numerical weather prediction (NWP) models, shortwave radiation is not a state variable, but a diagnostic variable. In other words, NWP models originally had not been designed for solar radiation forecasting.
WRF-Solar is an enhancement of the WRF model for a more accurate simulation of the aerosol-cloud-radiation interplay. In the recent years, the weather research and forecasting (WRF) model has been augmented and specifically adapted for better modelling the interaction of aerosols, clouds, and radiation 1,2. Numerous improvements in the parameterisation schemes of the WRF model have been implemented in WRF 3,4. This allows to largely reduce the forecast error of solar irradiance, especially in clear sky situations.
What is the WRF-Solar Ensemble Prediction System (EPS)?
WRF-Solar EPS is an extension to the WRF-Solar components of the WRF model. It introduces stochastic perturbations in the parameterisation variables that are most relevant for solar radiation arriving at the ground. These variables have been identified with tangent linear models. The stochastic perturbation methods in this state-of-art forecasting tool are especially chosen to account for uncertainties of representation in cloud, aerosol, and radiation processes 5. A high-quality ensemble prediction system like WRF-Solar EPS enables us to identify and quantify solar energy resources, as each ensemble member depicts a possible future state. The ensemble spread can be used to assess the range and uncertainty of solar power predictions and lead to improved probabilistic solar irradiance forecasting.
This interactive plot shows an example of global horizontal irradiance (GHI) as predicted by WRF-Solar EPS for Lindenberg (Germany) on 4 March 2022. WRF is set up to run on two domains: One with a horizontal grid spacing of 9 km, and the other one with a grid spacing of 3 km. The spread of the 5-member ensemble differs from one domain to the other. The observations originate from DWD’s Open Data portal.
Multi-layer cloud cover forecasts
A NWP model is a translation of physical equations into computer code. As processes in the atmosphere range from seconds to days and nanometer to kilometer ranges, weather models include many estimations, approximations, and omissions. The model is always limited by its resolution, which defines the smallest and most fleeting processes that are resolved. WRF allows to nest domains, meaning a nested high-resolution domain is initialised inside a domain with coarser grid spacing. Therefore, even with comparably small computational costs, a regional high-resolution forecast can be achieved and fine-scale cloud and convection processes can be computed by the model.
The animation shows an example of a WRF cloud cover forecast (high-level, mid-level, low-level clouds) in Central Europe with the same two domain setup. The right domain is nested into the left domain, meaning that it is not just a simple zoom. All physical processes are actually computed on the higher resolution and the boundary conditions are given by the other domain. The initial and boundary conditions for the domain on the left are given by the Global Forecast System (GFS), operated by NOAA. Note the difference between the two domains in terms of small-scale clouds. The WRF-Solar modifications of the parameterisation schemes lead to much more realistic high-resolution cloud fields.
Cloud mask comparison: WRF vs. satellite
Assessing the ability of a weather model to correctly predict a future state is referred to as verification. Especially with cloud predictions, quantifying the so-called forecast skill on a spatial level is essential to gauge the model performance. Here at Reuniwatt, we are computing a cloud mask from WRF and WRF-Solar predictions and can compare it to a satellite-based cloud mask. Those remotely sensed observations help to create a hit-or-miss statistic on which we can base different metrics and forecast skill calculations.
The figure shows an example of a WRF cloud mask forecast at 3 km grid spacing compared to a satellite-derived cloud mask. The difference plot reveals areas in which clouds are either present or absent in both WRF and the satellite, as well as the presence of clouds in either one, WRF or satellite.
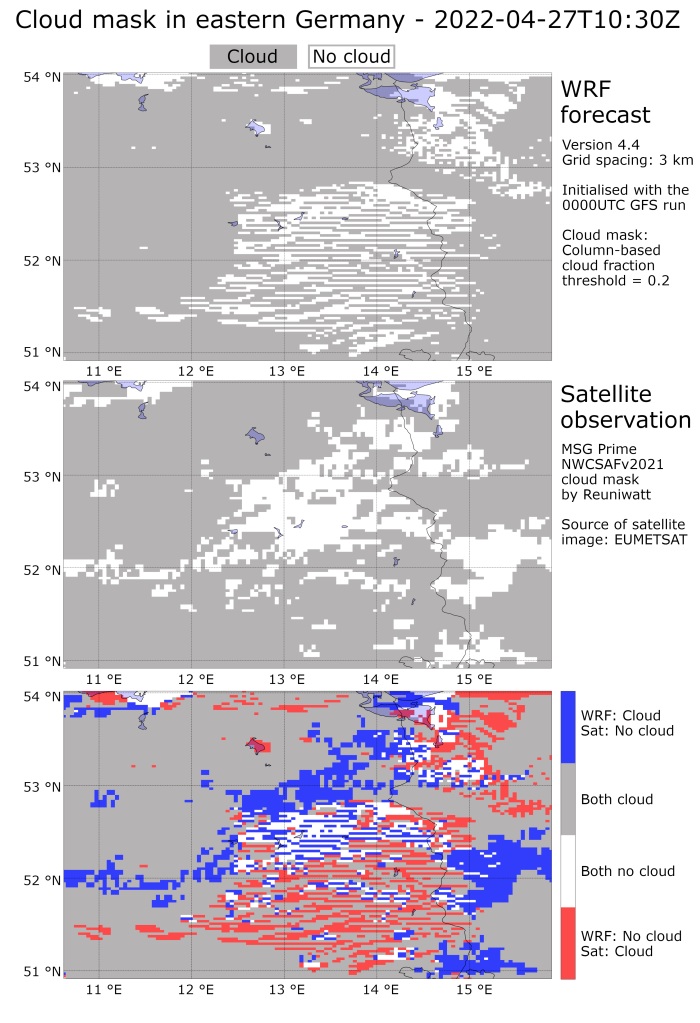
References
- Haupt, S. E., Kosovic, B., Jensen, T. L., Lee, J., Jimenez Munoz, P., Lazo, J. K., … Hinkleman, L. (2016). The Sun4Cast® Solar Power Forecasting System: The Result of the Public-Private-Academic Partnership to Advance Solar Power Forecasting (No. NCAR/TN-526+STR). doi:10.5065/D6N58JR2.
- Jimenez, P.A., J. P. Hacker, J. Dudhia, S. E. Haupt, J. A. Ruiz-Arias, C. A. Gueymard, G. Thompson, T. Eidhammer and A. Deng, 2016a: WRF-Solar: Description and Clear-Sky Assessment of an Augmented NWP Model for Solar Power Prediction. Bull. Amer. Met. Soc.. 97, 1249-1264. doi:10.1175/BAMS-D-14-00279.1.
- Ruiz-Arias, J.A., J. Dudhia and C. A. Gueymard, 2014: A simple parameterization of the short-wave aerosol optical properties for surface direct and diffuse irradiances assessment in a numerical weather model. Geosci., Model Devel., 7, 1159-1174.
- Xie, Yu, M. Sengupta, J. Dudhia, 2016: A Fast-sky Radiation model for Solar applications (FARMS): Algorithm and performance evaluation. Solar Energy, 135, 435-445.
- J. -H. Kim et al., “The WRF-Solar Ensemble Prediction System to Provide Solar Irradiance Probabilistic Forecasts,” in IEEE Journal of Photovoltaics, vol. 12, no. 1, pp. 141-144, Jan. 2022, doi: 10.1109/JPHOTOV.2021.3117904.